Plenary Keynote (Tues Sept 27)
Parthasarathy Ranganathan
VP/technical Fellow, Google
Make computing count: some grand opportunities for testing
Biography: Partha Ranganathan is currently a VP, technical Fellow at Google where he is the area technical lead for hardware and datacenters, designing systems at scale. Prior to this, he was a HP Fellow and Chief Technologist at Hewlett Packard Labs where he led their research on systems and data centers.
Abstract: Moore’s law is slowing down, stressing traditional assumptions around cheaper and faster systems every year. At the same time, growing volumes of data, smarter edge devices, and new, diverse workloads are causing demand for computing to grow at phenomenal rates. In this talk, we will discuss the trends shaping the future computing landscape, with a specific focus on the role of testing — for correctness, agility, and performance — and some grand challenges, and opportunities, for the field.
Wednesday Keynote
John Shalf
Lawrence Berkeley National Labs
The future of High Performance Computing Beyond Moore’s Law
Biography: John Shalf is Department Head for Computer Science at Lawrence Berkeley National Laboratory, and recently was deputy director of Hardware Technology for the DOE Exascale Computing Project.
Abstract: The next decade promises to be one of the most exciting yet in the further evolution of computing. There are a number of developments that will change how we will compute in 10 years: the foreseeable end of Moore’s law will lead to the exploration of new architectures and the introduction of new technologies in HPC; the rapid progress in machine learning in the last decade has led to a refocus of HPC towards large scale data analysis and machine learning; the feasibility of quantum computing has led to the introduction of new paradigms for scientific computing; meanwhile 30 billion IOT devices will push advances in energy efficient computing and bring an avalanche of data. I would like to compare the situation to a Cambrian explosion: the change in computing environment has helped creating a wide and complex variety of “organisms” that will compete for survival in the next decade. The HPC community will have to deal with this complexity and extreme heterogeneity, and decide what ideas and technologies will be the survivors. In this talk, I will talk about emerging strategies such as heterogeneous integration (advanced packaging) that are playing out across the industry to continue to extract performance from systems and make predictions of where things are going for 2025-2030.
Thursday Keynote
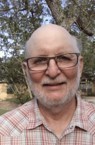
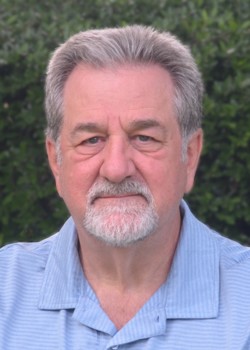
Grady Giles, Mike Bienek, & Tim Wood
AMD
What did we learn in 120 years of DFT and test?
Biography: Grady Giles, Mike Bienek, and Tim Wood are all members of the DFX team at AMD, with a combined 120+ years of experience in the industry.
Abstract: This interview-style discussion will feature three industry veterans whose careers have followed (and propelled) the growth in our field. We plan to reflect on how our industry has evolved, how this conference has reflected and driven that evolution, what lessons were learned, and what we can expect (and make happen) next. Along the way, we’ll share some anecdotes, tell some stories, brag about some accomplishments, and humbly give some advice on things we found out the hard way (so that you don’t have to).
Visionary Talk (Wednesday)
Tim Cheng
The Hong Kong University of Science and Technology
Ultra Low-Power AI Accelerators for AIoT –
Compute-in-memory, Co-Design, and Heterogeneous Integration
Biography: Tim Cheng is currently Vice-President for Research and Development at Hong Kong University of Science and Technology (HKUST) and Chair Professor jointly in the Departments of ECE and CSE.
Abstract: We will give an overview of the objectives and some recent progress in designing ultra low-power AI accelerators for supporting a wide range of AIoT devices with powerful embedded intelligence. Specifically, we will discuss the roles of emerging memory and compute-in-memory for data-centric computing, application-specific co-design framework supporting light-weight deep learning which integrates neural network (NN) search, hardware-friendly NN compression and NN-aware architecture design for iterative co-optimization, as well as the critical role of 3D integration of processors and memory arrays for power, performance and size.